The BDD Industry Consortium investigates state-of-the-art technologies in computer vision and machine learning for automotive applications. Our multi-disciplinary center is housed at the University of California, Berkeley and is directed by Professor Trevor Darrell, Faculty Director of PATH, Professor Kurt Keutzer and Dr. Ching-Yao Chan. The BDD consortium partners with private industry sponsors and brings faculty and researchers together from multiple departments and centers to develop new and emerging technologies with real-world applications in the automotive industry. Research is proposed by UC Berkeley faculty and approved by a BDD advisory board made up of faculty and sponsor representatives. Although dramatic progress has been made in the field of computer visioning, many of these technologies and theories have yet to carry over to the automotive field. Thus, the need and driving force behind the Berkeley DeepDrive Center.
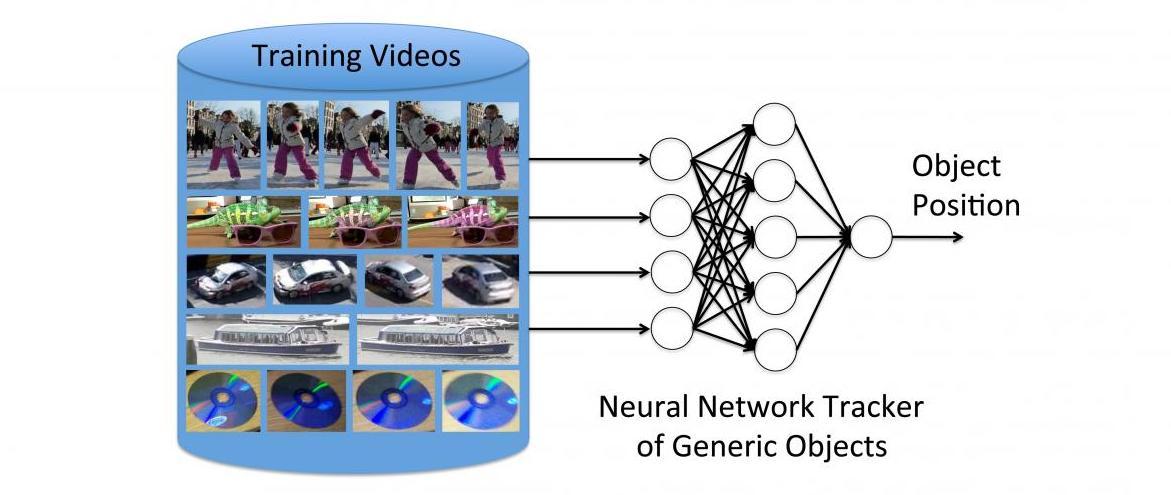
Deep Learning Reinforcement: By watching many videos of moving objects, the team’s new tracker learns the relationship between appearance and motion that allows it to track new objects at test time. This tracker thus establishes a new framework for tracking in which the relationship between appearance and motion is learned offline in a generic manner.
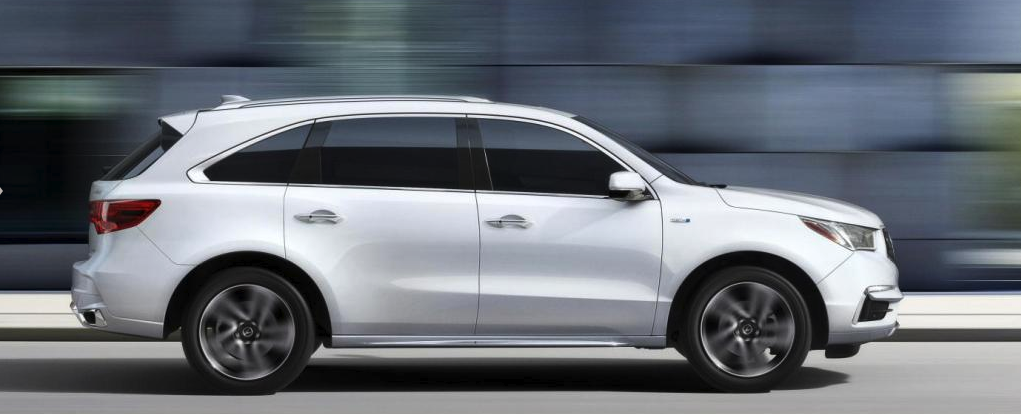
Cross-modal Transfer Learning: This project seeks to transfer models for vision tasks like object detection, segmentation, fine-grained categorization, and pose-estimation trained using large-scale annotated RGB datasets to new modalities with no or very few such task-specific labels.
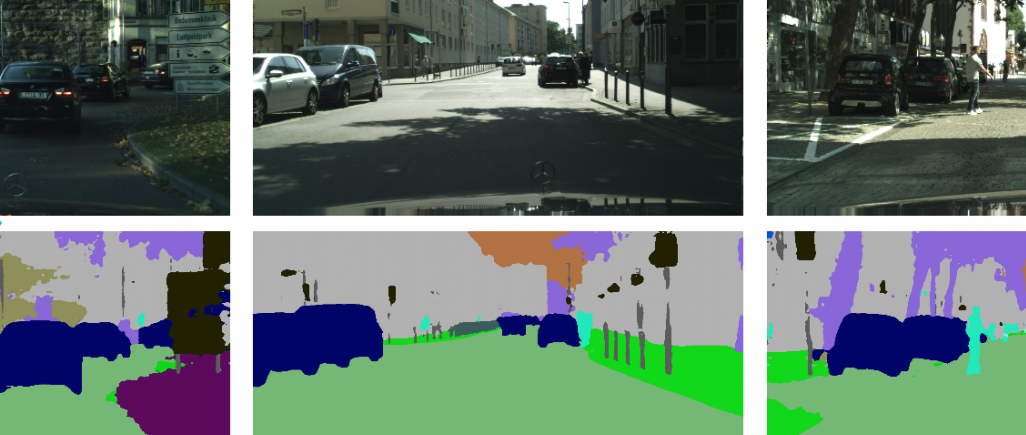
Clockwork FCNs for Fast Video Processing: The team will use a novel, frame asynchronous “clockwork” convnet, which pipelines processing over time with different update network rates at different levels of the semantic processing hierarchy, significantly reducing frame processing time with little or no degradation in performance.